Over the past two years dozens of newsrooms around the world have crafted policies and guidelines on how their editorial staff can or should — or cannot or should not — use artificial intelligence tools.
Those documents are tacit acknowledgement that AI, particularly generative AI like chatbots that can produce images and news stories at a keystroke, may fundamentally change how journalists do their work and how the public thinks about journalism.
Generative AI tools are based on large language models, which are trained on huge amounts of existing digital text often pulled from the web. Several news organizations are suing generative AI maker OpenAI for copyright infringement over the use of their news stories to train AI chatbots. Meanwhile, The Atlantic and Vox Media have signed licensing deals allowing OpenAI access to their archives.
Despite the litigation, some news organizations have used generative AI to create news stories, including the Associated Press for simple coverage of company earnings reports and college basketball game previews.
But others that have dabbled in AI-generated content have faced scrutiny for publishing confusing or misleading information, and the utility of generative AI in journalism is not obvious to everyone.
“The reality is that AI models can often prepare a decent first draft,” Julia Angwin, longtime tech reporter and newsroom leader, wrote recently in a New York Times op-ed. “But I find that when I use AI, I have to spend almost as much time correcting and revising its output as it would have taken me to do the work myself.”
To gain insight on what the future of AI and journalism might look like — and where the industry’s biggest challenges are — I reached out to Angwin, who has reported for The Wall Street Journal and ProPublica and in 2020 launched the award-winning nonprofit newsroom The Markup, which, among other things, covered recent AI developments.
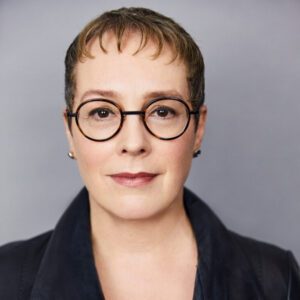
In early 2023 Angwin left The Markup and founded Proof News, a nonprofit news outlet that uses the scientific method to guide its investigations. Angwin is also a 2023-2024 Walter Shorenstein Media and Democracy Fellow at Harvard Kennedy School’s Shorenstein Center on Media, Politics and Public Policy, where The Journalist’s Resource is housed.
Social media creators and trust in news
During her time at the Shorenstein Center, Angwin interviewed a panel of social media creators to find out what journalists can learn from how creators and influencers share information and build trust with audiences. This summer, Angwin will publish a discussion paper on the findings.
One important way social media creators build trust is by directly engaging with their audiences, she found.
At the same time, some news organizations have turned away from direct audience engagement online.
“Newsrooms have, for all sorts of legitimate reasons, turned off the comments section because it’s hard to moderate,” Angwin says. “It also does mean that there’s a feeling from the audience that traditional news is less accountable, that it’s less responsive.”
AI in journalism
Angwin is not optimistic that generative AI will be useful to journalists, though AI tools are “totally legit and accepted” for reporting that includes statistical analysis, she says. But Angwin points to several concerns for the future, including that the use of copyrighted content to train generative AI systems could disincentivize journalists from doing important work.
Here are a few other highlights from our conversation about journalistic trust and the future of AI in journalism:
- The news business isn’t ready. Competing in an information ecosystem with generative AI that creates plausible sounding (but sometimes untrue) text is a new frontier for news organizations, which will have to be even more attentive in showing audiences the evidence behind their reporting.
- To gain trust, journalists need to acknowledge what they don’t know. It’s OK for journalists not to know everything about a topic they’re covering or story they’re pursuing. In published work, be upfront with audiences about what you know and areas you’re still reporting.
- When covering AI tools, be specific. Journalists covering AI topics need to know the types of AI tools out there — for example, generative versus statistical versus facial recognition. It’s important to clearly explain in your coverage which technology you are talking about.
The interview below has been edited for length and clarity.
Clark Merrefield: Some commentators have said AI is going to fundamentally change the internet. At this point it would be impossible to disentangle journalism and the internet. How would you characterize this moment, where AI is here and being used in some newsrooms? Is journalism ready?
Julia Angwin: Definitely I’d say we’re not ready. What we’re not ready for is the fact that there are basically these machines out there that can create plausible sounding text that has no relationship to the truth.
AI is inherently not about facts and accuracy. You’ll see that in the tiny disclaimer at the bottom of ChatGPT or any of those tools. They are about word associations. So for a profession that writes words that are meant to be factual, all of a sudden you’re competing in the marketplace — essentially, the marketplace of information — with all these words that sound plausible, look plausible and have no relationship to accuracy.
There’s two ways to look at it. One is we could all drown in the sea of plausible sounding text and lose trust in everything. Another scenario is maybe there will be a flight to quality and people will actually choose to go back to these mainstream legacy brand names and be like, “I only trust it if I saw it, you know, in the Washington Post.”
I suspect it’s not going to be really clear whether it’s either — it’s going to be a mix. In an industry that’s already under a lot of pressure financially — and, actually, just societally because of the lack of trust in news.
[AI] adds another layer of challenge to this already challenging business.
CM: In a recent investigation you found AI chatbots did a poor job responding to basic questions from voters, like where and when to vote. What sorts of concerns do you have about human journalists who are pressed for time — they’re on deadline, they’re doing a thousand things — passing along inaccurate, AI-generated content to audiences?
JA: Our first big investigation [at Proof News] was testing the accuracy of the leading AI models when it came to questions that voters might ask. Most of those questions were about logistics. Where should I vote? Am I eligible? What are the rules? When is the deadline for registration? Can I vote by text?
We took these questions from common questions that election officials told us that they get. We put them into leading AI models and we rated their responses for accuracy. We brought in election officials from across the U.S. So we had more than two dozen election officials from state and county levels who rated them for accuracy.
And what we found is they were largely inaccurate — the majority of answers and responses from the AI models were not correct as rated by experts in the field.
You have to have experts rating the output because some of the answers looked really plausible. It’s not like a Google search where it’s like, pick one of these options and maybe one of them will be true.
It’s very declarative: This is the place to vote.
If you already knew the answer, then maybe you should have just written the sentence yourself.
Or, in one ZIP code, it said there’s no place for you to vote, which is obviously not true.
Llama, the Meta [AI] model, had this whole thing, like, here’s how you vote by text: There’s a service in California called Vote by Text and here’s how you register for it. And it had all these details that sounded really like, “Oh, my gosh! Maybe there is a vote-by-text service!”
There is not! There is no way to vote by text!
Having experts involved made it easier to really be clear about what was accurate and what was not. The ones I’ve described were pretty clearly inaccurate, but there were a lot of edge cases where I would have probably been like, “Oh, it seems good,” and the election officials were like, “No.”
You kind of already have to know the facts in order to police them. I think that is the challenge about using [AI] in the newsroom. If you already knew the answer, then maybe you should have just written the sentence yourself. And if you didn’t, it might look really plausible, and you might be tempted to rely on it. So I worry about the use of these tools in newsrooms.
CM: And this is generative AI we’re talking about, right?
JA: Yes, and I would like to say that there is a real difference between generative AI and other types of AI. I use other types of AI all the time, like in data analysis — decision trees and regressions. And there’s a lot of statistical techniques that sort of technically qualify as AI and are totally legit and accepted.
Generative AI is just a special category and made of writing text, creating voice, creating images, where it’s about creation of something that humans used to only be able to create. And that is where I think we have a special category of risk.
CM: If you go to one of these AI chatbots and ask, “What time do I need to go vote and where do I vote?” it’s not actually searching for an answer to those questions, it’s just using the corpus of words that it’s based on to create an answer, right?
JA: Exactly. Most of these models are trained on data sets that might have data up until 2021 or 2022, and it’s 2024 right now. Things like polling places can change every election. It might be at the local school one year, and then it’s going to be at city hall the next year. There’s a lot of fluidity to things.
We were hoping that the models would say, “Actually, that’s not something I can answer because my data is old and you should go do a search, or you should go to this county elections office.” Some of the models did do that. ChatGPT did it more consistently than the rest. But, surprisingly, none of them really did it that consistently despite some of the companies having made promises that they were going to redirect those types of queries to trusted sources.
The problem is that these models, as you described them, they’re just these giant troves of data basically designed to do this are-these-words-next-to-each-other thing. When they rely on old data, either they were pulling up old polling places or they’re making up addresses. It was actually like they made up URLs. They just kind of cobbled together stuff that looked similar and made up things a lot of the time.
CM: You write in your founder’s letter for Proof News that the scientific method is your guide. Does AI fit in at all into the journalism that Proof News is doing and will do?
JA: The scientific method is my best answer to try to move on from the debate in journalism about objectivity. Objectivity has been the lodestar for journalism for a long time, and there’s a lot of legitimate reasons that people wanted to have a feeling of fairness and neutrality in the journalism that they’re reading.
Yet it has sort of devolved into what I think Wesley Lowry best describes as a performative exercise about whether you, as an individual reporter, have biases. The reality is we all have biases. So I find the scientific method is a really helpful answer to that conundrum because it’s all about the rigor of your processes.
Basically, are your processes rigorous enough to overcome the inherent bias that you have as a human? That’s why I like it. It’s about setting up rigorous processes.
Proof is an attempt to make that aspect the centerpiece. Using the scientific method and being data driven and trying to build large sample sizes when we can so that we have more robust results will mean we will do data analysis with statistical tools that will qualify as AI, for sure. There’s no question that will be in our future, and I’ve done that many times in the past.
I think that is fine — as I think it’s important to disclose those things. But those tools are well accepted in academia and research. Whenever I use tools like that, I always go to experts in the field, statisticians, to review my work before publishing. I feel comfortable with the use of that type of AI.
I do not expect to be using generative AI [at Proof News]. I just don’t see a reason why we would do it. Some of the coders that we work with, sometimes they use some sort of AI copilot to check their work to see if there’s a way to enhance it. And that, I think, is OK because you’re still writing the code yourself. But I don’t expect to ever be writing a headline or a story using generative AI.
CM: What is a realistic fear now that we’re adding AI to the mix of media that exists on the internet?
JA: Generative AI companies, which are all for-profit companies, are scraping the internet and grabbing everything, whether or not it is truly publicly available to them.
I am very concerned about the disincentive that gives for people to contribute to what we call the public square. There’s so many wonderful places on the internet, like Wikipedia, even Reddit, where people share information in good faith. The fact that there’s a whole bunch of for-profit companies hoovering up that information and then trying to monetize it themselves, I think that’s a real disincentive for people to participate in those public squares. And I think that makes a worse internet for everyone.
As a journalist, I want to contribute my work to the public. I don’t want it to be behind a paywall. Proof is licensed by Creative Commons, so anyone can use that information. That is the best model, in my opinion. And yet, it makes you pause. Like, “Oh, OK, I’m going to do all this work and then they’re going to make money off of it?” And then I’m essentially an unpaid worker for these AI companies.
CM: You’re a big advocate of showing your work as a journalist. When AI is added to that mix, does that imperative become even more critical? Does it change at all?
JA: It becomes even more urgent to show your work when you’re competing with a black box that creates plausible text but doesn’t show how it got that text.
One of the reasons I founded Proof and called it Proof was that idea of embedding in the story how we did it. We have an ingredients label on every story. What was our hypothesis? What’s our sample size?
That is really how I’m trying to compete in this landscape. I think there might be a flight to well-known brands. This idea that people decide to trust brands they already know, like the [New York] Times. But unfortunately, what we have seen is that trust in those brands is also down. Those places do great work, but there are mistakes they’ve made.
My feeling is we have to bring the level of truth down from the institution level to the story level. That’s why I’m trying to have all that transparency within the story itself as opposed to trying to build trust in the overall brand.
My feeling is we have to bring the level of truth down from the institution level to the story level.
Trust is declining — not just in journalistic institutions but in government, in corporations. We are in an era of distrust. This is where I take lessons from the [social media] creators because they don’t assume anyone trusts them. They just start with the evidence. They say, here’s my evidence and put it on camera. We have to get to a level of elevating all the evidence, and being really, really clear with our audiences.
CM: That’s interesting to go down to the story level, because that’s fundamentally what journalism is supposed to be about. The New York Times of the world built their reputation on the trust of their stories and also can lose it based on that, too.
JA: A lot of savvy readers have favorite reporters who they trust. They might not trust the whole institution, but they trust a certain reporter. That’s very similar to the creator economy where people have certain creators they trust, some they don’t.
We’re wired as humans to be careful and choose with our trust. I guess it’s not that natural to have trust in that whole institution. I don’t feel like it’s a winnable battle, at least not for me, to rebuild trust in giant journalistic institutions. But I do think there’s a way to build trust in the journalistic process. And so I want expose that process, make that process as rigorous as possible and be really honest with the audience.
And what that means, by the way, is be really honest about what you don’t know. There’s a lot of false certainty in journalism. Our headlines can be overly declarative. We tend to try to push our lead sentences to the max. What is the most declarative thing we can say? And that is driven a little bit by the demands of clickbait and engagement.
But that overdetermination also alienates the audience when they realize that there’s some nuance. One of the big pieces of our ingredients label is the limitations. What do we not know? What data would we need to make a better determination? And that’s where you go back to science, where everything is iterative — like, the idea is there’s no perfect truth. We’re all just trying to move towards it, right? And so we build on each other’s work. And then we admit that we need someone to build on ours, too.
CM: Any final thoughts or words of caution as we enter this brave new world of generative AI and journalism, and how newsrooms should be thinking about this?
JA: I would like it if journalists could work a little harder to distinguish different types of AI. The reality is there are so many kinds of AI. There’s the AI that is used in facial recognition, which is matching photos against known databases, and that’s a probability of a match.
There’s then the generative AI, which is the probability of how close words are to each other. There’s statistical AI, which is about predicting how a regression is trying to fit a line to a data set and see if there’s a pattern.
Right now everything is conflated into AI generally. It’s a little bit like talking about all vehicles as transportation. The reality is a train is really different than a truck, which is really different than a passenger car, which is really different than a bicycle. That’s kind of the range we have for AI, too. As we move forward journalists should start to distinguish a little bit more about those differences.
Expert Commentary